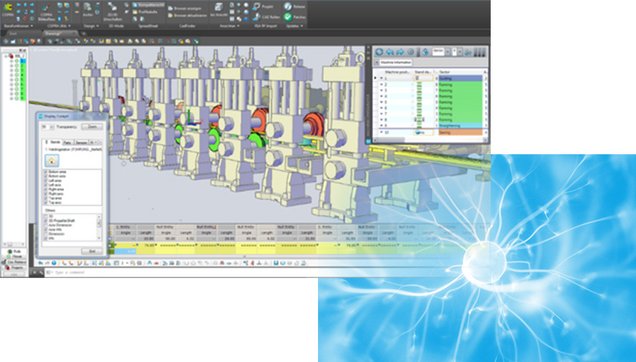
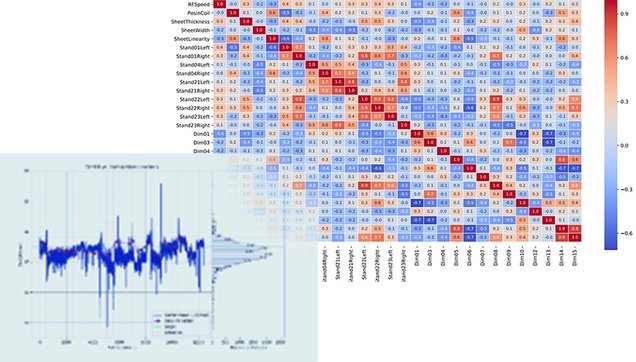
AI meets roll forming
Use of artificial intelligence (AI) also in profile production? With the granting of patent EP 3618981 (“rollforming unit with sensor-rollforming frame”) it became official: data M's latest COPRA technology combines intelligent software with the rollforming mill and sensors. This enables, for example, the use of assistance systems in production. data M's technology works independently of the respective machine manufacturer. It can also be integrated into existing production systems (retrofitting).
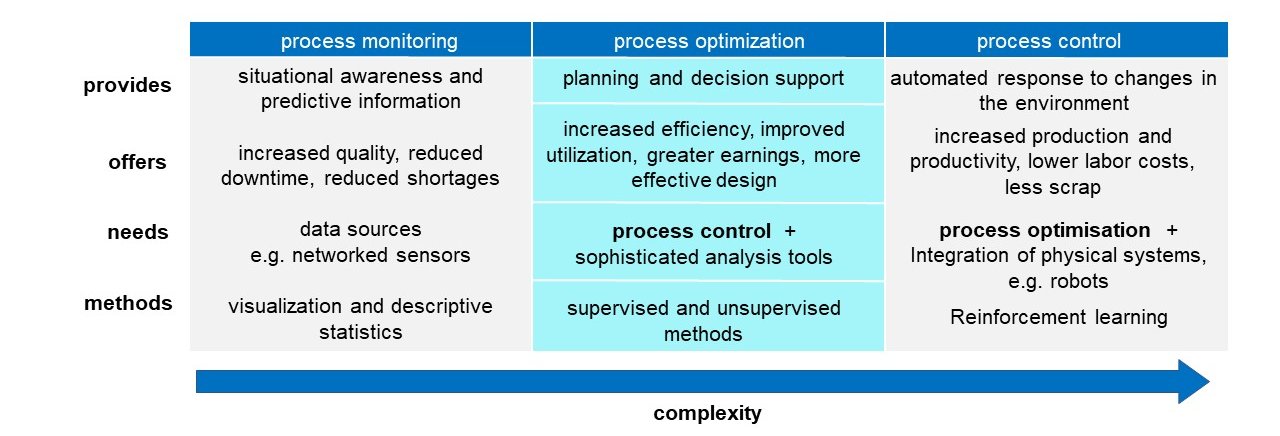
Artificial intelligence
The basic idea of artificial intelligence is the independent learning of the rules that a program uses to make decisions, e.g. by recognizing patterns in data sets. This distinguishes an AI-based program from a traditional one, in which the decision-making rules are given by a human. AI-based programs are therefore algorithms that can learn knowledge and make probability-based predictions. The human provides the necessary data and a suitable framework, after which the program adapts the rules accordingly. The result is faster pattern recognition by the AI than would be possible by humans.
What is mostly understood by AI today is the sub-area of machine learning (machine learning), in which the following three approaches are currently dominant:
- Supervised Learning
- Unsupervised Learning
- Reinforcement Learning
In the case of supervised learning, data sets that are labeled (ie outputs that are already defined for the inputs of the training data set) are passed to the algorithm so that it can, for example, classify new input data according to the recognized patterns. In unsupervised learning, the training data set is not labeled and the goal is more often pure pattern recognition or grouping of the data. Reinforcement learning uses the principle of reward and punishment in order to maximize reward through trial and error.
Figure 1 shows possible areas of application for these three methods in production processes.
For each of these methods, regardless of the area of application, the requirement of qualitatively and quantitatively high-quality datasets applies, since this is the only way models can be trained with sufficient precision.
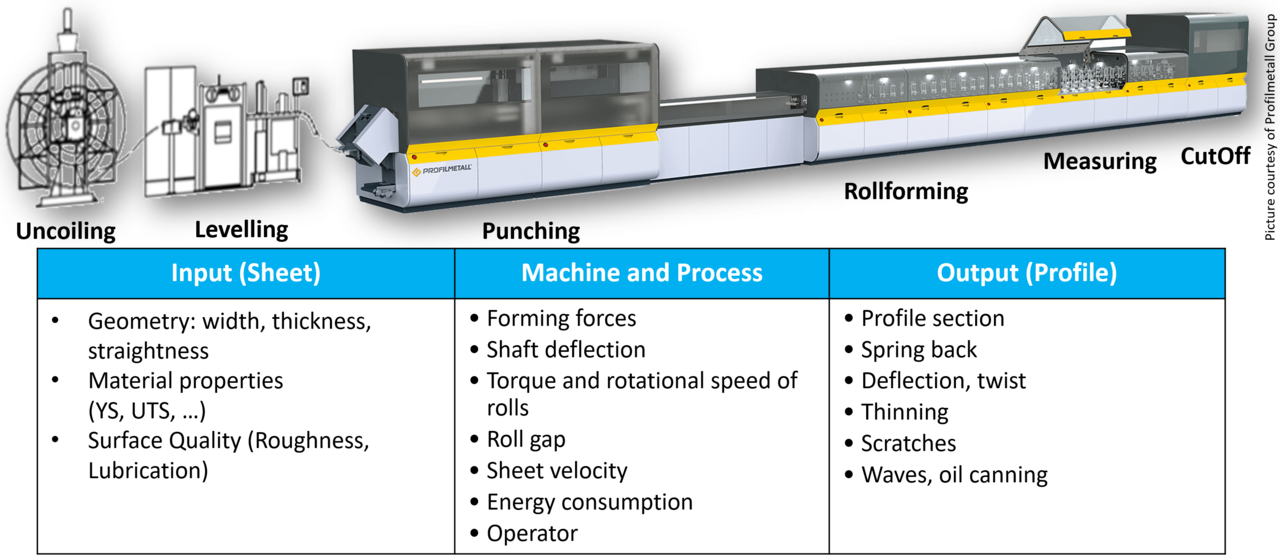
Data collection and data M's preliminary work in IntWal17
As mentioned in the "Artificial Intelligence" section, the basic requirement for meaningful AI applications is a high-quality data set. In production technology, this means determining the parameters of the production process and then recording them with suitable sensors.
data M has already been able to demonstrate its expertise in this area of data collection, for example in the government-funded project "IntWal17". In this project, which started in 2017, the process parameters of the roll forming process were determined, as can be seen in Figure 2.
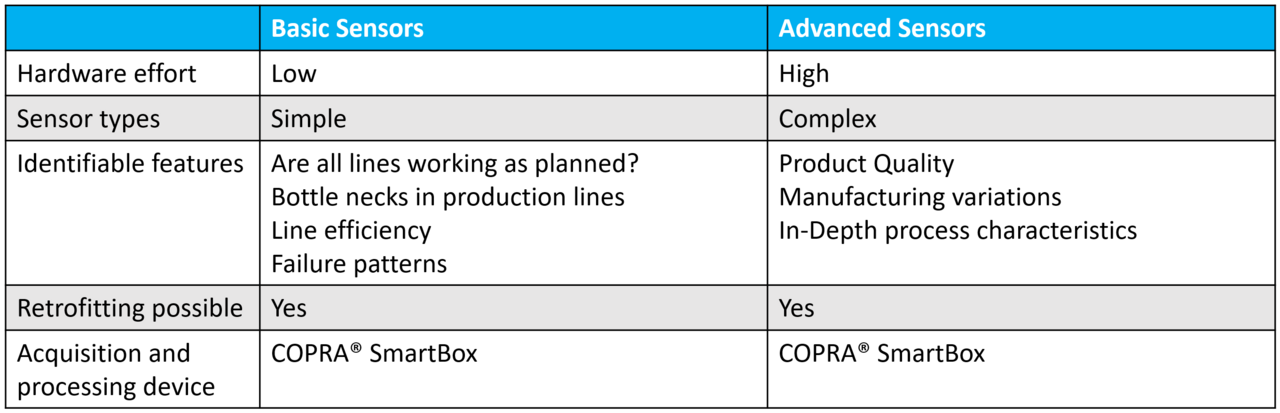
In order to measure these parameters and thus create a data set suitable for AI applications, data M developed a sensor system and an edge device.
The developed sensor system can be divided into the groups "Basic" and "Advanced". The "Basic" group includes sensors that are easy to install, such as friction wheels and light barriers, while the "Advanced" group contains more complex sensors, for example for profile geometry measurement. Table 1 compares the two groups.
The connection of the sensors was implemented in the form of the COPRA SmartBox, a universally applicable edge device that can provide the collected data via OPC UA. In addition, a first pre-processing and visualization of the data takes place in the COPRA SmartBox. Sensor data can be sent to any database system via the integrated OPC UA interface, be it a local database or a cloud-based system.
With the system presented, data M enables a clean data pool for modern AI applications, which are increasingly occurring in today's production facilities.
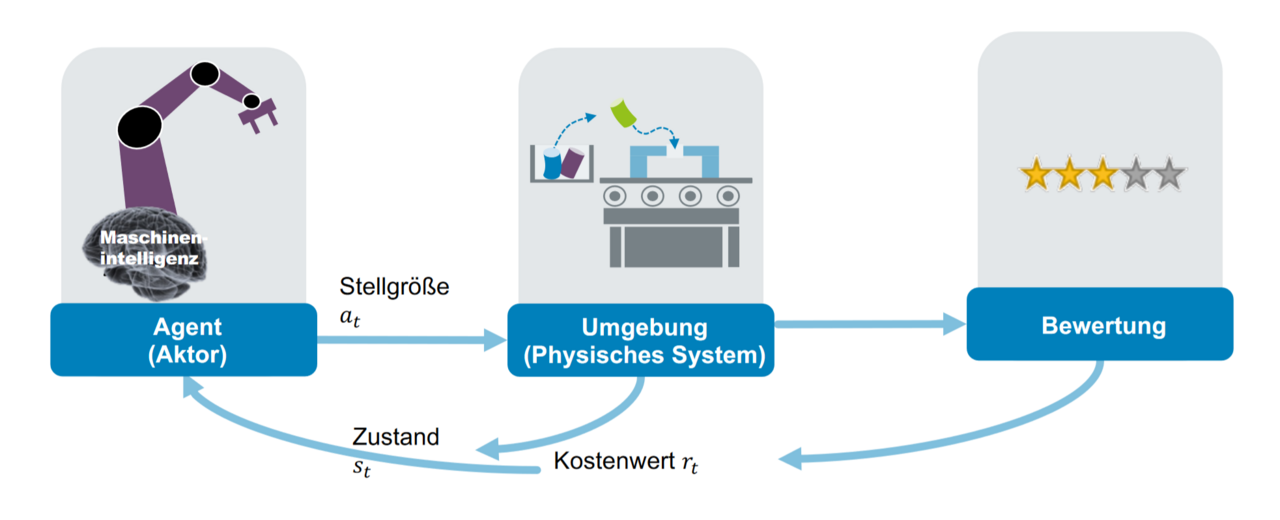
Areas of application in roll forming
Looking specifically at roll forming and the benefits this industry can derive from the large-scale collection of production data, possible applications include:
- process monitoring
- anomaly detection
- assistance systems
In principle, precise process monitoring does not require any complex evaluation tools, but can still provide considerable added value for the user. Prepared and visualized information about downtimes, production utilization and component quality increases process efficiency and thus the user's profit.
Unforeseen maintenance and repair work has a negative effect on process efficiency but can be, however, minimized with AI-supported anomaly detection. A negative influence on the competitive situation can thus be avoided. The principle of anomaly detection is based on recognizing those patterns in the measured parameters that lead to machine failures. By recognizing these failures at an early stage, response measures can be initiated in good time, such as servicing the machine during a planned downtime. In addition to this detection, an adjustment of the order control is possible through a suitable feedback, whereby delays in delivery can be avoided.
Another promising area of application for AI, or specifically machine learning, relates to assistance systems for production optimization and control . The optimization of production includes both the reaction to anomalies already described and the principle of offering decision-making aids in planning and production with the help of machine learning approaches. Thinking this principle through to the end leads to an application in production control, in which the system automatically reacts to changes in external factors. With the help of reinforcement learning, for example, an actor can be trained to adapt control variables in the physical system so that the resulting consequences receive the best possible evaluation, as shown in Figure 3 (VDMA 2019).
Conclusion
In summary, the possibilities of artificial intelligence in production technology are diverse, but not yet fully mature. In the past, data M laid the foundation for successful AI applications in roll forming and is now constantly working to advance this technology.
VDMA (2019): Leitfaden Selbstlernende Produktionsprozesse – Einführungsstrategie Reinforcement Learning in der industriellen Praxis [25.05.2022].